Demand Estimation with Large Product Sets: Using Machine Learning to Reduce Estimation Bias
April 11 2019
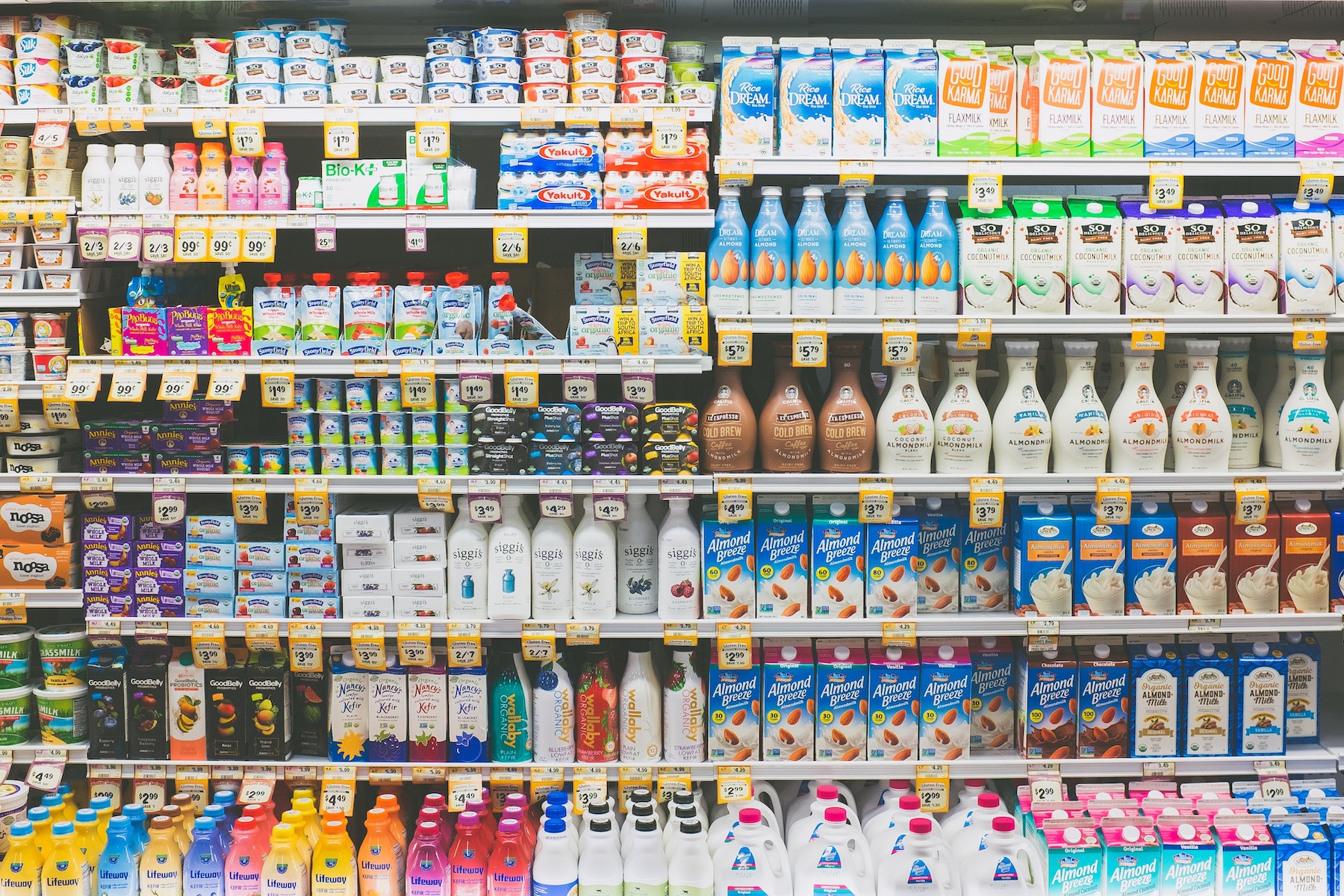
Status: Working paper
Abstract
Even though consumers face a vast variety of product options in online retailing, they usually only consider a limited number of alternatives for purchase. Traditional demand models, arising from situations in which a limited number of alternatives are available, assume that consumers consider all available products, which can lead to biased coefficient estimates in online settings. To limit this bias, we propose a method that explicitly incorporates consumers' consideration sets into the demand estimation process. Specifically, we leverage consumers' online clickstream data and infer consumers' consideration set distribution by applying a machine learning algorithm, namely, the graphical lasso model. This model allows us to estimate the correlation of consideration set inclusion among different products in a scalable way. Then we combine this consideration set distribution with a multinomial logit model of consumer choice. We use a series of numerical experiments to demonstrate that the proposed model can recover the true demand parameters with less bias than the traditional model. We also show that the proposed model is flexible enough to incorporate possible correlation between the consideration set and preference parameters (e.g. price coefficient). We then apply the proposed model to a real dataset and find that the traditional model overestimates the price elasticity compared with the proposed model.
Keywords: Demand Estimation, Consideration Set, Online Retailing, Graphical Lasso Model, Machine Learning, Clickstream Data
[Download Article]